EP1S40B956C5 Altera, EP1S40B956C5 Datasheet - Page 624
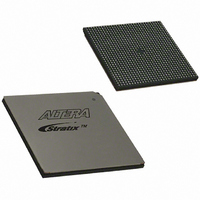
EP1S40B956C5
Manufacturer Part Number
EP1S40B956C5
Description
IC STRATIX FPGA 40K LE 956-BGA
Manufacturer
Altera
Series
Stratix®r
Datasheet
1.EP1S10F484I6N.pdf
(864 pages)
Specifications of EP1S40B956C5
Number Of Logic Elements/cells
41250
Number Of Labs/clbs
4125
Total Ram Bits
3423744
Number Of I /o
683
Voltage - Supply
1.425 V ~ 1.575 V
Mounting Type
Surface Mount
Operating Temperature
0°C ~ 85°C
Package / Case
956-BGA
Lead Free Status / RoHS Status
Contains lead / RoHS non-compliant
Number Of Gates
-
Available stocks
Company
Part Number
Manufacturer
Quantity
Price
Part Number:
EP1S40B956C5
Manufacturer:
ALTERA/阿尔特拉
Quantity:
20 000
- Current page: 624 of 864
- Download datasheet (11Mb)
Matrix Manipulation
7–46
Stratix Device Handbook, Volume 2
Two-Dimensional Filtering & Video Imaging
FIR filtering for video applications and image processing in general is
used in many applications, including noise removal, image sharpening to
feature extraction.
For noise removal, the goal is to reduce the effects of undesirable,
contaminative signals that have been linearly added to the image.
Applying a low pass filter or smoothing function flattens the image by
reducing the rapid pixel-to-pixel variation in gray levels and, ultimately,
removing noise. It also has a blurring effect usually used as a precursor
for removing unwanted details before extracting certain features from the
image.
Image sharpening focuses on the fine details of the image and enhances
sharp transitions between the pixels. This acts as a high-pass filter that
reduces broad features like the uniform background in an image and
enhances compact features or details that have been blurred.
Feature extraction is a form of image analysis slightly different from
image processing. The goal of image analysis in general is to extract
information based on certain characteristics from the image. This is a
multiple step process that includes edge detection. The easiest form of
edge detection is the derivative filter, using gradient operators.
All of the operations above involve transformation of the input image.
This can be presented as the convolution of the two-dimensional input
image, x(m,n) with the impulse response of the transform, f(k,l), resulting
in y(m,n) which is the output image.
The f(k,l) function refers to the matrix of filter coefficients. Because the
matrix operation is analogous to a filter operation, the matrix itself is
considered the impulse response of the filter. Depending on the type of
operation, the choice of the convolutional kernel or mask, f(k,l) is
different.
a larger image.
y m n
y m n
Figure 7–26
=
=
f k l
k
=
N
–
N
l
shows an example of convolving a 3
=
N
–
N
x m n
f k l x m k n l –
–
Altera Corporation
September 2004
3 mask with
Related parts for EP1S40B956C5
Image
Part Number
Description
Manufacturer
Datasheet
Request
R

Part Number:
Description:
CYCLONE II STARTER KIT EP2C20N
Manufacturer:
Altera
Datasheet:

Part Number:
Description:
CPLD, EP610 Family, ECMOS Process, 300 Gates, 16 Macro Cells, 16 Reg., 16 User I/Os, 5V Supply, 35 Speed Grade, 24DIP
Manufacturer:
Altera Corporation
Datasheet:

Part Number:
Description:
CPLD, EP610 Family, ECMOS Process, 300 Gates, 16 Macro Cells, 16 Reg., 16 User I/Os, 5V Supply, 15 Speed Grade, 24DIP
Manufacturer:
Altera Corporation
Datasheet:

Part Number:
Description:
Manufacturer:
Altera Corporation
Datasheet:

Part Number:
Description:
CPLD, EP610 Family, ECMOS Process, 300 Gates, 16 Macro Cells, 16 Reg., 16 User I/Os, 5V Supply, 30 Speed Grade, 24DIP
Manufacturer:
Altera Corporation
Datasheet:

Part Number:
Description:
High-performance, low-power erasable programmable logic devices with 8 macrocells, 10ns
Manufacturer:
Altera Corporation
Datasheet:

Part Number:
Description:
High-performance, low-power erasable programmable logic devices with 8 macrocells, 7ns
Manufacturer:
Altera Corporation
Datasheet:

Part Number:
Description:
Classic EPLD
Manufacturer:
Altera Corporation
Datasheet:

Part Number:
Description:
High-performance, low-power erasable programmable logic devices with 8 macrocells, 10ns
Manufacturer:
Altera Corporation
Datasheet:

Part Number:
Description:
Manufacturer:
Altera Corporation
Datasheet:

Part Number:
Description:
Manufacturer:
Altera Corporation
Datasheet:

Part Number:
Description:
Manufacturer:
Altera Corporation
Datasheet:

Part Number:
Description:
CPLD, EP610 Family, ECMOS Process, 300 Gates, 16 Macro Cells, 16 Reg., 16 User I/Os, 5V Supply, 25 Speed Grade, 24DIP
Manufacturer:
Altera Corporation
Datasheet: